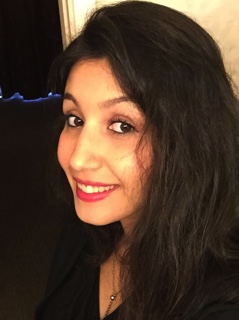
Mitigating Algorithmic Discrimination in Machine Learning
AI and machine learning tools are being used with increasing frequency for decision making in domains that affect peoples’ lives such as employment, education, policing and loan approval. These uses raise concerns about biases and algorithmic discrimination and have motivated the development of fairness-aware mechanisms in the machine learning (ML) community. In this talk, I will show how to measure bias and define fairness and why this is a challenging task. Then, I will present three categories of techniques to ensure fairness in different stages of the ML pipeline. I will conclude my talk with takeaways, open questions and future directions towards building a trustworthy ML system.